In the wake of the 2022 crypto winter, the massive hype around a blockchain-based internet and the vision of a decentralised, read-write-own version of the web began to fade. Web3 was no longer cool, as startups flaunting ‘Web3’ in their vision statements found themselves out of favour with investors. As Web3 venture funding declined over consecutive quarters, the “metaverse” — another overused buzzword — experienced a parallel decline in both popularity and interest.
Echoing the crypto bear market of 2018, virtual land prices fell, and the dream of living the “Ready Player One” life remained just that – a dream, despite Mark Zuckerberg’s Meta with its mission “to bring the metaverse to life and help people connect, find communities and grow businesses”, struggled to breathe life into this market.
Then came June 2023 and Apple’s announcement of the “Apple Vision Pro”, a ‘revolutionary spatial computer’ promising unprecedented possibilities of blending digital content with physical space — allowing users to do things in ways never before possible. Although VR/AR wearables were not groundbreaking innovations, they hinted at a new way of experiencing the internet, a potential portal to the metaverse.
Ironically, Apple’s stock dipped more than usual post-announcement (averaging a 0.3% decline during WWDC keynotes since 2013), dashing hopes of a metaverse renaissance. While the new pair of goggles doesn’t herald the dawn of a new internet, I argue that the metaverse isn’t built for consumers, at least not at first. While we’ve enjoyed exciting virtual experiences, like virtual Justin Bieber concerts, digital art showcases by national museums, and avatar-based fashion weeks, it remains uncertain whether these use cases have instilled the confidence needed for mass adoption of this future — particularly when the price tag of a new-age, mixed-reality device starts at $3,499.
Hence, for the metaverse to truly become the world we live, work and play in, organisations must discover its value before consumers do. How? Through digital twins.
The enterprise metaverse is a thing
Digital twins are set to become the foundation of a metaverse for enterprises. As the barrier between physical and digital environments starts to blur, organisations will experience a transformation in how they operate, manage and innovate — one that involves replicating assets, people, processes, transactions and other work-related facets in massive virtual, interconnected realms. Enabled by VR/AR, AI and other emerging tech, digital twins offer immersive, data-driven experiences to enterprises — allowing, for example, product managers to simulate real-time customer benefits upon making app-feature changes or senior leaders to make smarter decisions through dynamic simulations of growth trajectories based on multivariate analysis of individual investment options.
The metaverse isn’t just a place where user avatars socialise, show off cool skins and purchase branded digital products; it could be a place where enterprises thrive using personalised digital replicas of their organisation — digital twins. These twins redefine value delivery and transform experiences, ushering in a new era of decision-making accuracy and innovation.
Here is a scenario for senior leaders, managers and executives to contemplate.
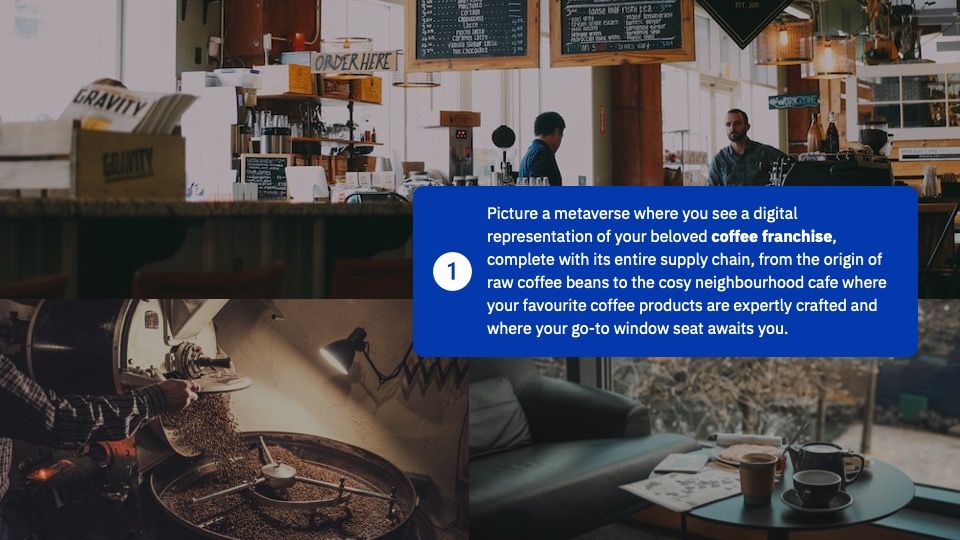
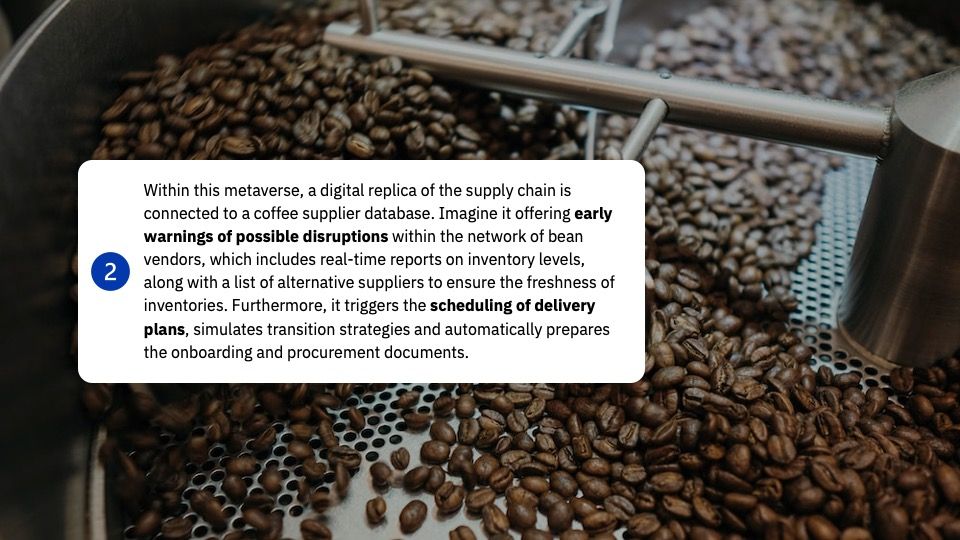
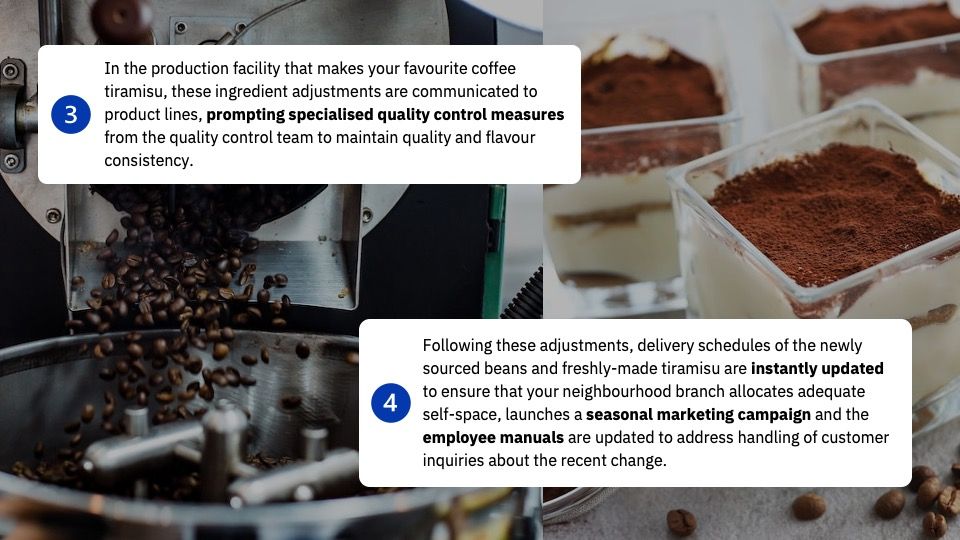
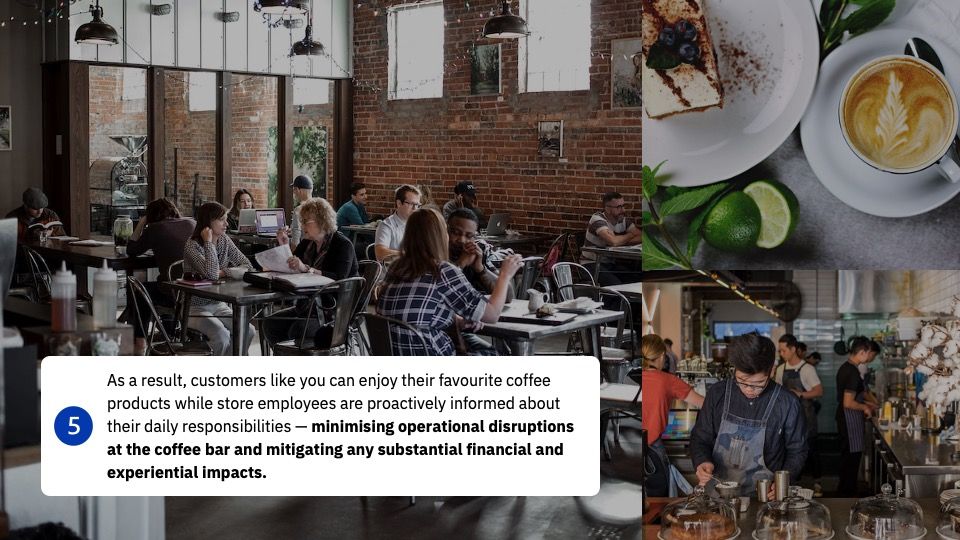
This is the promise of an enterprise metaverse. The combination of multiple digital twins formed on cross-organisational data updated in real-time, advanced simulation and predictive capabilities, and visualisation models creates the foundation of a digital, immersive environment that replicates everything from products, production lines, processes and employees. Here, organisations can augment new experiences and elevate data-driven decision-making through dynamic simulations.
It is crucial to emphasise that true value lies not in creating the enterprise metaverse but in the process of developing digital twins that generate iterative improvement insights that can be effectively applied to both existing assets and possibly future ones.
Anatomy of a digital twin
Think of digital twins as a virtual representation of real-world objects, entities or systems as they operate within their unique environments. While there are many definitions, keep one key distinction in mind: a basic visualisation or digital representation of a physical asset, even your CAD drawing, is not a digital twin.
At its core, a digital twin holds data connected to reliable sources distributed throughout the organisation. It acts as a constant feed of real insights, ensuring that operating algorithms and models remain continuously updated to mirror changes to the physical asset or process as they unfold. Digital twins are inherently dynamic and can be personalised to represent different parts within or related to an organisation.
For example, a product twin embodies a virtual representation of products, like a pizza delivery app, that reflects the entire product life cycle — from the design and engineering phase to post-launch operations, where users are placing pizza delivery orders. Beyond this, there exist other archetypes of digital twins, including network twins, infrastructure twins, parts twins, and systems and process twins. These can be further disassembled to represent the minutest facets of the organisation, such as a warehouse, sales process, inventory levels, and call centre — supporting complex AI use cases in even the lowest levels.
A communications layer serves as the bridge that links cross-functional data, paving the way for predictions, simulations, and recommendation models powered by machine learning and analytics platforms. These platforms build upon a foundational data layer and culminate in a visualisation layer, offering seamless accessibility and shared application use, ultimately driving value for enterprises.
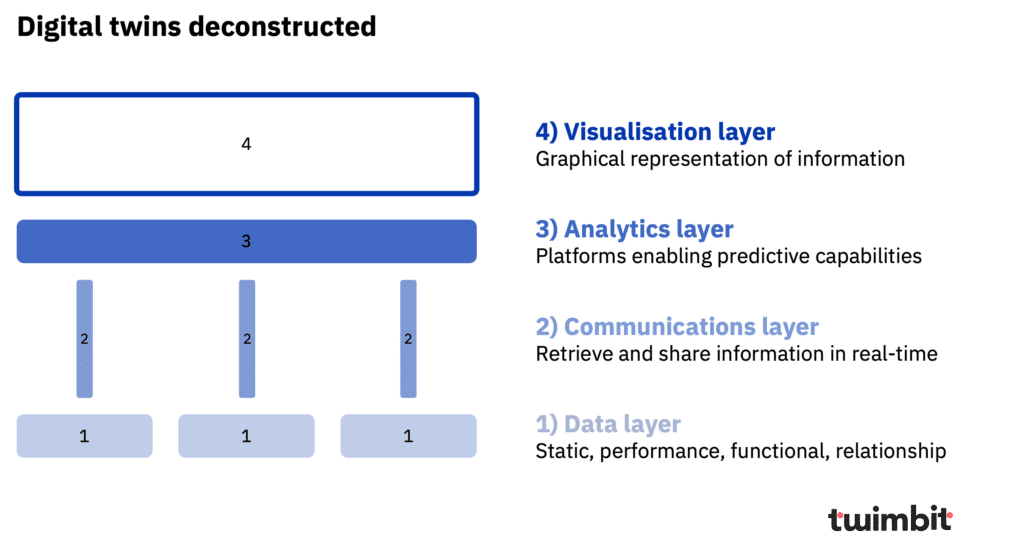
Digital twins have the potential to accelerate the time to market for new applications, enabling real-time iterations and design optimisation as the development process takes place digitally or, at least before it happens physically. Notably, this digital replication streamlines the product development cycle by eliminating the need to gather and structure raw data at the outset, resulting in improved product quality, cost efficiency and productivity management.
Alternatively, consider the concept of digital twins of a customer (DToC), which takes a step beyond traditional 360-degree customer profiles, typically used to help organisations know more about who they are serving and built on the aggregation of transaction, preference and relationship data. Instead, a customer digital twin uses probabilistic and iterative models to simulate, emulate and anticipate customer behaviour — especially valuable in times of uncertainty and changing habits. For example, a retailer can leverage insights derived from digital twins of in-store customers to make strategic adjustments to store layouts, product placements, inventory levels and adjust frontline staff availability. The result is an enhanced overall shopping experience, increased revenue driven by larger average basket sizes, and higher operational margins.
Digital twins: stronger when connected
While perfecting one digital twin can yield significant benefits, true transformation occurs when we establish connections between two or more digital twins. This interconnected network, akin to replicating the intricate web of a knowledge graph, enables the collaboration power of multiple digital twins to simulate complex, often concealed, relationships — unlocking profound insights and paving the way for advanced use cases beyond the capabilities of a single twin.
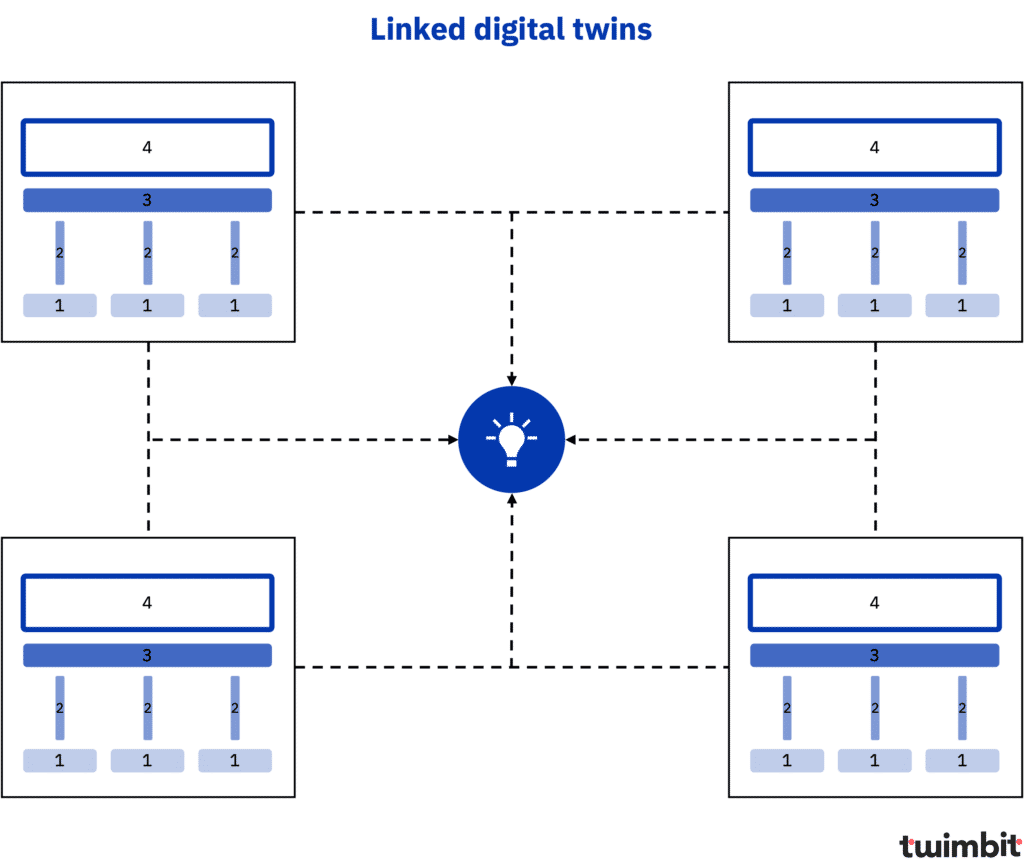
Consider the coffee franchise scenario mentioned earlier. By linking its supply chain twin with digital representations of customers, numerous retail outlets across the country, its mobile ordering application, and frontline employees, a wealth of new sales insights can be generated. This personalised, interlinked system allows for the simulation of various store scenarios and the prediction of customer experiences across diverse journeys. Ultimately, it guides smarter decision-making and facilitates seamless management, resulting in a more agile and responsive organisation.
Planning for digital twins
To dive into the realm of digital twins, organisations must lay a foundation of digital maturity. This means having the right data infrastructure in place, as well as access to high-quality data suitable for both testing and real-world deployment. If leaders find themselves uncertain about where to begin, conducting a digital maturity assessment can serve as an illuminating compass. It reveals existing capabilities and sheds light on the gaps that demand additional infrastructure development.
The next pivotal step involves the careful selection of an apt use case for digital twins. Ideal candidates often emerge from dynamic environments that thrive on real-time optimisation. For a smooth start, it’s advisable to begin with a use case that strikes a balance — specific enough to be actionable but not overly complex. This approach allows organisations to methodically construct the entire digital twin framework.
In addition to this, it’s imperative to view the digital twin within the broader context of your digital strategy. Consider where it fits into the grander vision, how this capability can be wielded to gain a competitive edge in the marketplace, and, importantly, how this technology integrates with existing systems, data infrastructure, and processes. Is it a standalone solution, or does it need to interoperate with other technologies and architectures?
Selecting which digital twin to build first will require organisations to strategically evaluate which real-world asset or process holds the key to solving customers’ problems and exhibits reusability across high-priority domains closely tied to value creation. Moreover, organisations must weigh the feasibility of constructing the digital twin, factoring in aspects such as data availability, quality, and accessibility. Not every object or process is suitable for digital replication, and it’s essential to assess whether the investment in creating a digital twin is sensible from a financial standpoint.
Birth of the first twin
Once sound reasoning and commercial viability are sorted out, organisations should start assembling a data layer encompassing a range of cross-functional, high-quality, easily accessible, and reusable sets of structured and unstructured data. These datasets and parameters should be capable of representing the real-world asset or process with the utmost granularity to enhance real-world performance. To facilitate this process, data should be centred around customers rather than focused on sales or operational context, allowing deeper analysis of behavioural insights rather than transactional observations.
For instance, consider a data layer enriched with real-time information on inventory and buffer levels, inbound delivery schedules, supplier performance, warehouse capacity, and real-time utilisation rates. Such a layer not only streamlines sourcing activities but also serves as a definitive source of truth, laying the groundwork for continually evolving use cases.
To get started on the right foot, organisations should gather a diverse team of data engineers, data scientists and people from the business and IT functions. The cross-collaboration is crucial for designing solutions and supporting the initial infrastructure build-out. Additionally, having a proficient product manager with experience in MLOps and agile methodologies can assume a leadership role, orchestrating the team’s efforts and the creation of the digital twin. Furthermore, the team should be trained to precisely model physical assets using a physic-based approach — a process of using mathematical equations and simulations to replicate how the physical asset behaves under various conditions — especially when replicating complex products or facilities, where even subtle variations can have significant impacts.
Once the foundation is established with a capable team and a well-constructed data layer, organisations must act swiftly to integrate analytics platforms and capabilities that generate tangible insights for their teams. For instance, incorporating anomaly detection models and recommendation engines, harmonised with an iterative data feedback loop, can enhance operations quality control and in-app product suggestions. To elevate the predictive and prescriptive capabilities of digital twins, it’s crucial to integrate data layers with high-quality visualisations through AR/VR technology. This not only facilitates the simulation of new scenarios but empowers teams to deconstruct and reengineer entire processes, effectively transforming your data into a real digital twin.
As organisations expand their digital twin initiatives, they should consider additional factors in the post-build phase. The establishment of robust data governance structures requires a comprehensive framework that puts in place a set of practices to manage data throughout its lifecycle — to ensure data quality, security, compliance and accountability. A transparent process for identifying value and measuring organisational impact should be defined, facilitating the effective evaluation of digital twin investments versus the return on investment (ROI) derived from early digital twin use cases. By meticulously addressing these considerations, organisations can navigate the digital twin landscape with confidence and strategic precision, further making their case.
From twins to the metaverse
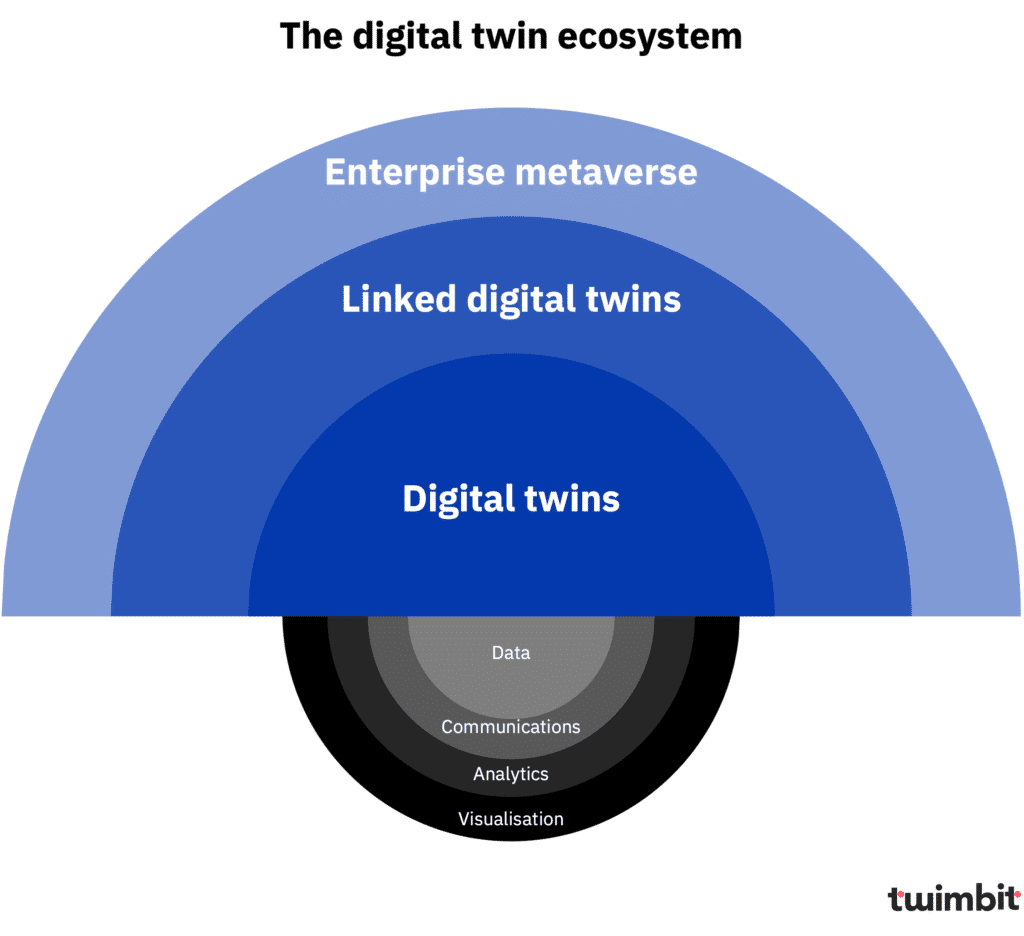
As organisations emerge from the pandemic, a profound shift in operational models is underway — particularly in asset-intensive industries such as manufacturing, logistics and healthcare. This transformation demands a holistic integration between both physical and digital replicas of assets and processes, together with predictive and prescriptive capabilities driven by AI. At the heart of this transformation are digital twins, redefining how organisations will innovate and deliver value.
The future of digital twins is nearly limitless, fuelled by continuous increases in processing power and cognitive abilities with advancements in chip technology and machine learning platforms. With the rise of AI and high-definition visualisation engines, use cases for digital twins in the enterprise metaverse are well within reach.
Today’s executives are not only starting to invest in digital twins but view the enterprise metaverse as fundamental to strategy, with almost two-thirds of leaders already beyond metaverse-related experimentation (Source: PwC 2022 US Metaverse Survey). This strategic shift lays the groundwork for a transformative era in which organisations across every industry will harness the full potential of data and AI, reshaping their operations and unlocking unprecedented innovation opportunities.
Looking ahead, we envision a future where digital twins serve the greater good of society. Consider the digital twin of planet Earth, empowered by models trained to simulate the governing laws of nature and predict our collective destiny. Although this visionary concept is currently under development, led by entities like the European Commission and NASA, it epitomises the boundless promise in this pivotal enabler of the enterprise metaverse: digital twins.